When using generative Artificial Intelligence (AI), the issues of bias and hallucinations in particular gain practical importance. These problems can arise both when using external AI tools (such as ChatGPT) and when developing your own AI models. So which data protection issues exist in relation to AI under the General Data Protection Regulation (GDPR) and what options are available to solve them?
What is bias in AI?
AI bias(es) are distorting effects in the development and use of AI. Particularly in machine learning (ML), a subcategory of AI, there often manifests a tendency of human bias and prejudice. AI bias occurs when an algorithm produces systematically biased results because of flawed assumptions in the ML process. Algorithms can thus, reveal and reinforce existing biases or even create new biases by placing trust in the distorted datasets.
For example, an algorithm for facial recognition could be trained in a way that it more easily recognizes men than women, because this type of data was more commonly used in training. In the automotive industry, for example, crash tests were long conducted using only dummies modeled on the male body and thus did not adequately take into account the special characteristics of women.
Another example involves job applications, where algorithms might reject photos with dark skin color and/or foreign names, even though the professional suitability could be objectively assessed to be equal or better on the basis of the available data. This means existing human bias can be mirrored or even reinforced by algorithms.
Bias can thus have a negative impact on people from minority groups. Discrimination caused by AI bias then in turn hinders equal opportunity and the biased condition is perpetuated.
In the case of so-called “Unconscious Bias” (unintentional distortion effects), it is difficult to recognize those effects.
Black-box problem
Bias in unstructured AI is reinforced by the so-called black-box problem. This occurs in certain forms of AI when the behavior or decisions of an AI system are not transparent or comprehensible to humans. In particular, this often makes it difficult to understand how a specific outcome was generated by the AI. The black box issue can arise in several areas of AI, including ML, neural networks, Large Language Models (LLM)/Natural Language Processing (NLP), and other complex AI models. The black-box problem becomes particularly relevant when AI systems are used in safety-critical sectors, such as autonomous vehicles, medical diagnostic systems, or financial decision-making.
Although significant progress has already been made in solving the black-box problem, it remains a challenge and can ultimately only be solved through cooperation between research, industry and regulatory authorities. The Data Protection Officer should always be involved at an early stage to evaluate existing requirements and possible solutions in the individual case.
So-called hallucinations in generative AI occur when the AI alleges or invents false facts (for example fake news), in particular, by presenting sources, contexts, or events that do not correspond to the truth or are contradictory. In this case, the processing of personal data is protected in particular by the GDPR and the right to rectification of data subjects applies.
Privacy implications
If personal data is being processed, opaque AI systems, AI bias, and AI hallucinations pose a threat to the protection of personal data. This is especially true when AI systems are not transparent. As training data for AI often requires a large amount of personal data (Big Data), it is often difficult to ensure comprehensibility for data subjects (for details on transparency requirements, see our blog post – Generative artificial intelligence and GDPR’s transparency obligations).
If no personal data is being processed, the GDPR does not apply. Therefore, it’s important to first assess the extent to which personal data is necessary for the specific AI application. If this is the case, for example, a legal basis is required and further requirements under the GDPR apply (among others, often, a data protection impact assessment (DPIA) will be necessary).
In the case of hallucinations the risk is, in particular, that sensitive personal data or trade secrets are falsely generated or disclosed. In addition, objectively false data can lead to data protection incidents and/or be misused by attackers.
The Black-Box problem further complicates the possibility of obtaining informed consent from users of an AI-based application, if such consent is required. Without transparency about how the personal data of affected individuals is used and the decisions made based on this data, consent, according to current standards, cannot generally be considered informed as per Art. 6(1)(a) in conjunction with Article 7(1) of the GDPR. A conceivable solution is to at least list the known criteria. In addition, the model of the so-called “broad consent” known from the research area could be transferred to AI systems.
Under the GDPR, the data subject also has the fundamental right not to be subject to a decision based solely on automated processing (including profiling), which has a legal effect vis-à-vis the data subject or significantly affects the data subject in a similar way.
What can be done to prevent bias?
To mitigate privacy implications, techniques for interpretable machine learning, the establishment of transparency standards for AI models, and the implementation of data protection laws and policies specific to AI and automated decision making are already being used in the research field as well as at regulatory level. Within the company itself, the known criteria should also be governed in a policy.
Further measures that can be taken to prevent or at least minimize bias and hallucinations in AI systems need to be evaluated on a case-by-case basis.
These include – with the involvement of the Data Protection Officer – in particular:
- Ensuring data quality and data diversity: When collecting data, it is crucial to ensure that the used data is of high quality, balanced and representative. Depending on the specific use case, the data must adequately cover different population groups, characteristics and perspectives. If bias is present, techniques such as data cleaning, weighting, or artificial expansion of the data can be utilized to ensure a more balanced representation.
- Promote diversity in development teams: Ideally, diverse teams of developers, data scientists, and subject matter experts with different backgrounds, genders, ethnicities, etc should be deployed. This allows different perspectives to be brought in and potential sources of bias to be better identified and uncovered.
- Principles of purpose limitation, necessity and data minimization: According to the GDPR, general principles such as purpose limitation (see our blog post on change of purpose for details (in German)), necessity and data minimization apply. Training data may generally only be collected and used to the extent that the data is actually necessary for the specific purpose.
- Use interpretable and transparent models: Using models that can explain how decisions are made can help to better understand and address bias. Where possible, preference should be given to interpretable models such as decision trees or linear models. Criteria and procedures for data collection, processing, and use in AI systems should be made transparent as far as individually possible and necessary. This will enable a certain degree of control and comprehensibility for the data subjects.
- Continuous monitoring and evaluation: AI systems should be regularly monitored and evaluated to ensure that no bias occurs or increases over time. In particular, this can be done by using metrics, testing and external reviews (audits). In addition, training data must be continuously monitored and checked for bias and discrimination (analysis/ monitoring). Furthermore, with regard to hallucinations, the AI results must be checked for accuracy and completeness, ideally by a human. To ensure accountability, this must also be documented.
- Technical-organizational measures: In particular, to prevent hallucinations, technical-organizational measures such as access restrictions and security measures to ensure integrity and confidentiality are advisable.
- Comply with internal policies and standards: The development and application of AI should ideally follow ethical guidelines and standards. Organizations should implement internal policies and processes to ensure that AI systems are used fairly, transparently and responsibly. The level of technical-organizational and regulatory measures taken should be individually regulated and communicated in a policy.
Challenges ahead for AI
It remains a challenge to counter bias and hallucinations in AI. Data protection requirements are only part of the solution and additional measures are required.
The individual solution depends in particular on the AI system used, the intended use, “the state of the art” (as referenced in GDPR Article 32(1)), and other regulatory requirements, such as under the proposed AI Act. After conducting a case-specific analysis, with a particular focus on the peculiarities of the AI system, it can be determined that, in practice and from a data protection perspective, everything currently feasible has been done.
Kirsten Ammon is an associate in Fieldfisher’s Hamburg office and advises on IT and data protection law, fintech and adtech, cloud computing, artificial intelligence, blockchain (especially smart contracts), e-commerce and legal tech.
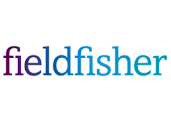