As cyber threats continue to evolve in complexity and frequency, the cybersecurity industry has made significant strides in bolstering endpoint defenses.
Leading Endpoint Detection and Response (EDR) platforms such as CrowdStrike Falcon, Microsoft Defender for Endpoint, and SentinelOne Singularity have become synonymous with robust endpoint security. These tools continuously monitor endpoint activity using a blend of signature-based detection, behavioral analytics, and machine learning algorithms to identify suspicious activities and novel attacks. They leverage cloud-based analysis for swift threat identification and response, ensuring real-time alerts for security teams.
The best defense isn’t just knowing what’s out there – it’s being prepared for what you don’t see coming.
Yet, despite these advancements, the industry has largely stagnated in one critical area: true anomaly detection.
Current detection methodologies
The current detection methodologies are still overly reliant on signature-based models, which inherently suffer from a fundamental flaw – they are reactive by design. Signatures are built upon prior knowledge of known attack techniques, meaning that zero-day exploits and novel attack methodologies remain significant blind spots. While behavioral analytics and machine learning capabilities have been introduced, they often play a secondary role, augmenting rather than replacing signature-based detection.
The result? Many EDR solutions still fall short of delivering proactive defense mechanisms capable of catching truly anomalous behavior before it escalates into a full-blown breach.
Data-driven anomaly detection
The problem lies in the gap between what EDR platforms claim to do and what they achieve in practice. True anomaly detection requires robust data analysis and machine learning models capable of understanding and contextualizing baseline “normal” behavior for endpoints, users, and systems. By identifying deviations from these baselines – rather than simply flagging known malicious behaviors – security tools can become predictive and proactive, detecting threats that do not rely on pre-logged attack patterns.
Unfortunately, many current tools lean heavily on configuration-driven rules rather than data-driven insights. This approach limits their ability to detect early-stage anomalies indicative of sophisticated attacks, such as lateral movement, privilege escalation, and data exfiltration.
Relying on configuration thresholds creates bottlenecks in detection times, leaving organizations vulnerable to advanced persistent threats (APTs) that deliberately operate beneath predefined thresholds.
Innovation is crucial
Innovation is crucial now to stay ahead of attackers who are increasingly leveraging zero-days, supply chain attacks, and even AI-driven malicious code. innovation in endpoint anomaly detection is not optional – it’s imperative. And here’s why.
Accelerating detection times
Current tools are often reactive, identifying breaches long after attackers have gained footholds. Anomaly detection powered by advanced machine learning can shrink the time from breach to detection, significantly mitigating potential damage.
Adapting to novel attacks
Attackers thrive on exploiting blind spots. By focusing on anomalous behavior rather than predefined rules, security solutions can evolve to detect novel and sophisticated attacks that traditional signature-based models miss.
Minimizing human error
Security teams are often overwhelmed by alert fatigue from misconfigured tools and false positives. Anomaly detection reduces noise by contextualizing alerts, ensuring teams focus on genuine threats rather than chasing shadows.
Improving breach response
Anomaly detection doesn’t just flag threats – it provides context and behavioral patterns that help teams respond more effectively, preventing breaches from escalating further.
The road ahead
Redefining endpoint detection to achieve meaningful progress, the industry must prioritize the development of tools that emphasize machine learning-driven anomaly detection over signature-reliant models. These tools should actively learn from endpoint data, adjust baselines dynamically, and provide security teams with actionable insights. By building smarter systems that focus on “unknown unknowns,” the next generation of endpoint solutions can move the industry from reactive to proactive defense.
Innovation in this space will also require collaboration between vendors, researchers, and enterprises to refine algorithms, improve training data quality, and deploy models capable of real-time anomaly detection at scale. Only then can organizations achieve the level of resilience needed to face the evolving threat landscape.
In summary
While tools like CrowdStrike Falcon, Microsoft Defender for Endpoint, and SentinelOne have set the benchmark for modern endpoint security, the heavy reliance on legacy methods like signature detection underscores a critical need for transformation.
Without true anomaly detection, organizations are fighting a losing battle against attackers who thrive on unpredictability.
The future of endpoint security lies in its ability to predict, detect, and neutralize threats before they cause harm. As cybercriminals grow more sophisticated, it’s time for the cybersecurity industry to rise to the challenge – investing in tools and methodologies that prioritize anomaly detection, machine learning, and data-driven defense. After all, the best defense isn’t just knowing what’s out there – it’s being prepared for what you don’t see coming.
Marc Brown serves in multifaceted executive roles as CEO, CMO, CPO, CRO, and Research. He is currently a member of the board and proposed director for Zayda Technologies. His extensive executive experience spans critical business functions, including marketing, product development, and revenue optimization, focusing on IT and OT/ICS cyber security, AI/ML products, software tools, embedded/real-time operating software, and middleware.
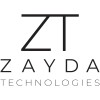