The significance of artificial intelligence is growing continuously. There are new developments in AI-based programs almost every day. It is not just private individuals who are experimenting with generative AI. It is now also present in many companies. Ideally it can optimise processes and help with certain selection decisions.
But the increasing visibility of AI also brings the question of the objectivity of AI-based decisions to the fore and there are reports of discrimination by AI.
Due to the basic manner of how AI works it is often not possible to trace back exactly how discriminatory decisions come about. There is no question that AI would be better and more useful if it were able to prevent discrimination and exclude human biases. However, in addition to the difficulty of tracing the origin of discriminatory decisions and preventing them as far as possible when programing and training AI, such discriminatory phenomena also pose challenges to the legal system as a means of combating injustice.
The German General Act on Equal Treatment (AGG, “General Equal Treatment Act”) and laws on data protection are only two of the possible regulatory means to prevent and combat discrimination by AI from a legal perspective. In addition, various approaches to regulating AI are being planned at the European level.
Evidence of AI discrimination
The consequence is, on the one hand, that the AI results are increasingly precise, but on the other hand, it is no longer possible to predict what AI will produce with any specific input.
In the past, there have been several reported cases of algorithms producing clearly discriminatory decisions. In 2018, for example, it became known that a major internet retailer was using an AI system that was supposed to assist in making decisions in the recruiting process. It was reported that this led to discriminatory hiring practices against women. The cause turned out to be a lack of quality in the software’s training data. In 2019, it was also announced that Apple Pay provided different credit limits for men and women. Women regularly received lower credit limits and were disadvantaged by Apple’s algorithm.
The Google Photos application labelled photos of Black people as gorillas (“Black people” is a self-designation and describes a social position affected by racism). “Black” is capitalised to make it clear that it is a constructed pattern of attribution and not a real characteristic attributable to skin color, see (only in German) Amnesty International glossary) and People of Color (“People of Color” is an international self-designation of/for people with experiences of racism, see (only in German) Amnesty International glossary).
Regardless of skin color, the algorithm also identified people as dogs in some cases. In the US, a software (COMPAS) which is used to help government agencies predict the likelihood of recidivism among offenders also caused a stir. The results of the software are used both in sentencing and in decisions on applications for early release. The software was repeatedly the subject of proceedings before the US Supreme Court because it predicted that black Americans and Americans of color were significantly more likely to be repeat offenders compared to white Americans (“white” is not a biological characteristic or a real skin colour, but a political and social construction, see (only in German) Amnesty International glossary).
These examples only exemplify different dimensions of discriminatory decisions by AI applications. The reasons for the discrimination by AIs are manifold, and it is often not possible in retrospect to trace the circumstances that led AI to one result or another.
Quality of training data sets
Discriminatory decisions can be inherent in the design of AI and in its training. A discriminatory conception is to be assumed above all when prejudices established in society are transferred into the algorithm or technical specifications lead to certain groups of people being treated differently from others. However, discrimination can also arise during training and when using AI. For example, a chatbot can be systematically “fed” with xenophobic conversations or views.
Even if the causes of discrimination by AI are manifold, it is at any rate clear that the quality of an AI decision depends significantly on the quality of the training data. Outdated or incomplete data sets can lead to incorrect and discriminatory results. AI can only learn from the data that is made available to it. Often, data sets that companies use for AI programmes are purchased and reused without any quality control, since the effort required for this would be comparatively high.
Facial recognition software, for example, is not inherently discriminatory. However, if it is trained predominantly with images of “white” people, it may not recognise or classify “non-white” people as well. This is less a result of the faulty design of the AI than of incorrectly selected training data.
AI can only learn from the data that is made available to it.
However, even if the data entered reflects demographic groups in equal parts, the results can still be distorted if, for example, the AI associates gender-specific characteristics of applicants predominantly with certain (stereotypical) positions and bases its decision on them. AI can also show discrimination if the developers select certain variables that are already discriminatory in themselves. The AI simply “learns” what it is “taught” and would therefore also base its results on discriminating variables.
What’s more, decisions by AI do not always have to be based on causal correlations. Humans also draw the wrong conclusions and this cannot be completely avoided when using AI either.
Transparency of machine decisions is often demanded. However, this is not so easy in practice. Self-learning algorithms are often so complex that it is hardly possible to understand what the decisions are based on. Companies also often have an interest in ensuring that such information is not made available to the public.
Ideally, however, AI will only learn from data that are correct and not inherently discriminatory. It is difficult to influence AI later when the data are being used, and it is therefore very susceptible to discriminatory decisions. Ideally, AI would be intelligent enough when it is being used to classify such information as discriminatory itself.
The General Act on Equal Treatment
Protection against discrimination has constitutional status in Germany (Article 3 German Basic Law (GG), “Basic Law”) and at European level (Article 21 and Article 23 Charter of Fundamental Rights (CFR)).
Against this background, the General Act on Equal Treatment – in addition to the implementation of various EU directives – fulfils the purpose of a concretisation of the constitutional mandate of equal treatment in ordinary statutory law. The General Act on Equal Treatment provides for individual legal protection against discrimination by non-governmental bodies, whereas Article 3 Basic Law only applies to unequal treatment in the relationship between the state and its citizens. The aim of the General Act on Equal Treatment as defined in section 1 is to prevent or eliminate discrimination, eg on the grounds of ethnic origin, gender, religion or belief, disability, age or sexual identity.
To achieve this goal, the General Act on Equal Treatment provides various rights for those affected, in particular a right of appeal (section 13), a right to refuse performance (section 14) and claims for compensation and damages (section 15).
AI in employment
In the material scope of application, the General Act on Equal Treatment distinguishes between the protection of employees (section 2 (1) nos. 1-4) and protection under civil law (section 2 (1) nos. 5-8). The corresponding prohibitions of discrimination are regulated in section 7 General Act on Equal Treatment, in the context of employment, and, among others, in section 19 General Act on Equal Treatment for routine business under civil law.
An example of use of artificial intelligence in context of employment is the Recruiting AI mentioned above, which automatically pre-filters applications based on preferred criteria as part of the applicant selection process. In the context of civil law, some examples where artificial intelligence is used include banks (in the context of creditworthiness assessment) and insurance companies (for risk assessment).
The applicability of the General Act on Equal Treatment for AI-supported decision-making processes is not prevented by the fact that, when AI systems are used, discrimination is not directly caused by a human decision. It is generally recognised in literature and case law that a legally relevant act by a person can also be carried out through a software-supported, automated process (eg in the context of liability for autofill suggestions by search engines that violate personal rights).
In such cases, the automation of the process is attributed as a technical aid, in the same way that activities by outsourced personnel are attributed to an employer. Therefore, the General Act on Equal Treatment is essentially technology-neutral; the scope of application therefore also extends to the use of AI.
Transparency of AI
The use of artificial intelligence does not so much test the law itself, but rather the enforcement of it, which comes down to the decision making processes of AI systems which is regularly described as a black box. Even if implementation of transparent algorithms in the sense of “explainable AI” is already being researched, it is questionable to what extent such models will be able to establish themselves in the future; in any case, “explainable AI” has not yet established itself as a technical standard.
This (still) existing system-imminent non transparency of artificial intelligence makes it difficult to check the reasons for decisions and makes it very difficult for those concerned to provide evidence, especially in the case of indirect discrimination within the meaning of section 3 (2) General Act on Equal Treatment, which is probably the most common form of discrimination by AI systems.
Direct discrimination (section 3 (1) General Act on Equal Treatment) is directly linked to one of the characteristics worthy of protection under section 1 General Act on Equal Treatment and is therefore easy to identify. In contrast, indirect discrimination is characterised by the fact that it results from seemingly neutral criteria or procedures, in that a person is disadvantaged in a particular way compared to another because of a reason mentioned in section 1 General Act on Equal Treatment (eg a job advertisement in which applicants are required to have German as their mother tongue, even though the job does not require that the applicant has excellent knowledge of German and certainly not that they have “German as a mother tongue”).
Indirect discrimination is characterised by the fact that it results from seemingly neutral criteria or procedures.
The distinction between these forms of discrimination involves a significant limitation of individual protection: if a legitimate objective is pursued using indirect discrimination and the means to achieve the objective are appropriate and necessary, there is a reason justifying the unequal treatment (section 3 (2) second half-sentence General Act on Equal Treatment). However, the General Act on Equal Treatment does not provide for the possibility of justifying direct unequal treatment.
The affected parties who in principle have the burden of proof under the General Act on Equal Treatment generally have the problem – even with unjustified discrimination based on a human decision – that transparent reasons are not provided for negative decisions (eg in the context of a job advertisement) and that there is also no general legal right to information.
Unjustified discrimination by AI
However, the black box of AI makes it factually impossible to prove unjustified unequal treatment. The provision of section 22 General Act on Equal Treatment under which the burden of proof is eased for the persons affected by presuming that the unequal treatment is based on one of the characteristics mentioned in section 1 General Act on Equal Treatment is also meaningless when artificial intelligence is used, due to the technical reasons already mentioned. The presumption of section 22 General Act on Equal Treatment only applies if the person concerned has succeeded in proving all of the criteria for the offence.
Even though the status quo described (still) lacks effective protection against unjustified discrimination by AI systems, it should be noted that the General Act on Equal Treatment as it exists already applies to such systems. Accordingly, those affected can generally fall back on the individual claims of the General Act on Equal Treatment (sections 15 (1), (2), and 21 (2)), the use of AI “just” makes it more difficult to enforce these claims.
The European legislator has recognised this issue and is attempting to address it through a comprehensive European legislative project, which, in particular, imposes transparency and disclosure obligations on operators.
Data protection
Data protection law also sets limits on the use of AI. The General Data Protection Regulation (GDPR) does not protect against discrimination directly. However, it provides strict guidelines for automated decision-making and can therefore help to prevent discriminatory decisions in certain scenarios.
Article 22 GDPR prohibits decisions that are detrimental which are based solely on automated processing of personal data. Article 22 GDPR reads: “The data subject shall have the right not to be subject to a decision based solely on automated processing, including profiling, which produces legal effects concerning him or her or similarly significantly affects him or her.”
The rationale behind the provision is that data subjects must not be made the mere object of algorithm-based automation. However, the rule only applies if the data processed is personal data. It is also sufficient if it is possible to draw conclusions about a natural person. However, if data is anonymised for training purposes, the GDPR does not apply.
Moreover, the provision only applies when a decision is solely the result of an automated processing operation. However, discrimination risks also arise in cases where AI is only used to prepare decisions and the final decision is made by a natural person. The prohibition under Article 22 GDPR only applies if the decision has legal effect in relation to the data subject, which can at least be assumed if the data subject’s legal status is changed (eg termination).
The GDPR pursues the protection of the right to self-determination with regard to the use of information and does not primarily serve to prevent discrimination.
It is already clear from the clearly defined scope of application of the GDPR, which presupposes the processing of personal data, that the GDPR is not the appropriate set of rules to prevent discriminatory AI decisions. The GDPR pursues the protection of the right to self-determination with regard to the use of information and does not primarily serve to prevent discrimination. The other restrictions in Article 22 GDPR also make it unsuitable to prevent discriminatory AI decisions.
Since machine learning often does not involve processing personal data, Article 5 GDPR, which sets out the principles of transparent and correct data processing, does not help either. Although there would be a certain appeal if the GDPR were to apply to discriminatory AI scenarios, as the GDPR has a considerable deterrent effect with high potential fines and could thus provide impetus in the right direction, it does not seem appropriate to have data protection supervisory authorities judge whether or not discrimination has occurred.
The fact that discrimination can occur in the context of automated processing must therefore be addressed by other regulations.
European legislation
On April 21, 2021, the EU Commission published a proposal laying down harmonised rules on artificial intelligence (draft AI Regulation) which aims, among other things, to complement existing Union law on non-discrimination.
“With specific requirements that aim to minimise the risk of algorithmic discrimination, in particular in relation to the design and the quality of data sets used for the development of AI systems complemented with obligations for testing, risk management, documentation and human oversight throughout the AI systems’ lifecycle.”
The draft takes a risk-based approach by differentiating between different levels of risk and setting corresponding specifications. The higher the potential risk, the higher the requirements for the AI system should be. Building on this principle, the draft provides for the prohibition of particularly dangerous AI systems (Article 5 draft AI Regulation), regulates provider and user obligations and defines binding requirements for high-risk AI systems (Article 8 ff. draft AI Regulation, especially with regard to transparency and documentation).
It can be assumed that the risk-based approach pursued with the current draft will remain in place even after the European legislative process for the AI Regulation is completed. This regulatory principle stems from the general principle of proportionality and has already proven its worth in the GDPR.
The higher the potential risk, the higher the requirements for the AI system should be.
There are severe sanctions for breaches of provisions of the AI Regulation (fines up to €30m ($32.9m) or 6% of the total annual global turnover, whichever is higher), which even exceed the fines under the GDPR.
The examples of use of artificial intelligence mentioned at the beginning (selection of applicants with the help of recruiting AI and in the context of creditworthiness checks by banks) do not constitute particularly dangerous, prohibited AI systems under the draft AI Regulation, but they are classified as high-risk AI systems (Article 6 (2) in conjunction with Annex III no. 4 (a), no. 5 (b) draft AI Regulation). As a result, extensive transparency and documentation obligations and data quality and data governance requirements are imposed on providers and users (Article 8 ff. draft AI Regulation):
- According to Article 13 draft AI Regulation, high-risk AI systems […] must be “designed and developed in such a way to ensure that their operation is sufficiently transparent to enable users to interpret the system’s output and use it appropriately.”
- In addition, Article 14 draft AI Regulation provides for human oversight of the systems, which aims at preventing or minimising risks to fundamental rights, among other things.
- In addition, the training, validation and test data sets of high-risk AI systems must meet the quality criteria set out in Article 10 (2) – (5) draft AI Regulation and in particular “be relevant, free of errors and complete”.
Documentation obligations
From this non-exhaustive list, the regulatory approach of the European legislation becomes clear, according to which, on the one hand, preventive measures are made binding in order to prevent discrimination through AI as far as possible, and on the other hand, through the planned transparency and documentation obligations for providers and users, the aim is (presumably) to make it easier for those affected by unequal treatment by AI to assert their claims.
However, the current concept of the draft regulation fails to achieve this aforementioned goal.
On the one hand, the draft does not provide access to the training, validation and test data sets used by the providers to the persons affected and create “unrestricted transparency“. Instead, only the “market surveillance authorities” will have direct access to this data in accordance with Article 64 draft AI Regulation. They are, however, subject to a confidentiality obligation under Article 70 draft AI Regulation.
The question of whether the proposed European legislation will actually improve the enforcement of rights for those affected therefore also depends to a considerable extent on the technical and financial resources of the “market surveillance authorities” ,which will be confronted with having to check large amounts of data and complex algorithms.
On the other hand, the European legislative proposal implies that those affected by unequal treatment by AI systems are usually also the users of the systems, which is not necessarily the case. For example, the requirements of Article 13 draft AI Regulation, which was already mentioned:
aims exclusively
“high-risk AI systems […] must be designed and developed in such a way to ensure that their operation is sufficiently transparent […]”
“to enable users to interpret the system’s output and use it appropriately.”
This requirement does not apply to third parties who are not users of the systems, but have experienced unequal treatment from the output. Although the general disclosure obligation under Article 52 draft AI Regulation also takes affected third parties into consideration, it is limited in that
“natural persons are informed that they are dealing with an AI system […],” which is already guaranteed by the GDPR.
Regulation must keep pace with technology
Artificial intelligence will without a doubt significantly influence the private, social and professional reality of life in the near future. This makes it all the more important that decisions made or supported by AI move society forward, not only in terms of the speed of decision-making, but also in terms of accuracy and impartiality.
Warnings about potentially biased recommendations from AI systems are just the beginning. Anti-discriminatory approaches must be actively considered when designing and training AI, and where discriminatory decisions or recommendations occur, legal means of defence should be available to those affected. In ratified law, the possibilities are limited because the regulations to date have not yet taken AI into account or considered it as a regulatory objective.
The European risk-based regulatory approach may set the course for effective law enforcement in this area by making the aforementioned preventive precautions binding and, at the same time, also addressing the opacity of the systems to actually enable state institutions to monitor the outcome. However, since the regulation of training data and the general transparency obligations offer considerable potential for discussion within the legislative process it is likely that there will be extensive revisions. In light of this, it is necessary to await and observe the development of this European legislative process.
Dr Lukas Hambel is an associate in the Hamburg office of CMS. He advises medium-sized and listed companies on IT law matters, with a focus on IT sector contracts. He also advises on complex IT outsourcing projects and the legal aspects of the introduction of ERP systems.
This article was co-authored by Annina Barbara Männig, who has now left CMS.
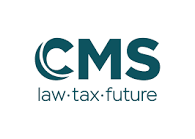