Red teaming is used by organizations to test the flaws and vulnerabilities present within the GenAI models, and the datasets used. It has its historical origins in the Cold War, when the US military prepared its forces by training them with simulated attacks from the Soviet Union by indicating its team as “red” while the US indicated its team as “blue”.
Since then, the use and purpose of red teaming has evolved and commercially is mainly used in the cybersecurity space, where organizations train cybersecurity systems through simulated cyberattacks. Red teaming is not only performed by corporations. Institutions in the EU also use its the protocols and procedures under the TIBER-EU framework.
With artificial intelligence (AI), red teaming has further developed in meaning and use, especially with the deployment of generative AI (GenAI) systems or general-purpose AI as per the EU AI Act. Red teaming is used to test the flaws and vulnerabilities present within the GenAI models, and the datasets used; specifically, red teams pressurize the general-purpose AI model through inputs to sieve out which prompts would generate malicious output.
Malicious output may not necessarily be limited to harmful content; it also includes content that constitutes copyright infringement (for example, plagiarism of text and pictures) or content that breaches data protection laws.
Companies may have standard operating procedures to detail the prompts that would result in malicious content and an action plan on dealing with such prompts. The implementation, non-implementation, and degree of implementation of red teaming within a business that offers its general-purpose AI model to other uses may result in a range of legal issues, which this article aims to discuss.
General-purpose AI
Red teaming aims to find flaws and prevent or reduce malicious output generated by the general-purpose AI model through rigorous adversarial testing and pressure. Product liability issues may arise when the general-purpose AI model still provides output that harms its users, notwithstanding the red teaming efforts of the provider of the general-purpose AI model. In this case, the question lies in the applicable standard on when red teaming is deemed to be sufficient to resolve the provider from product liability.
Generally, software – in which general-purpose AI models would fall under its ambit – are regulated by the recently revised EU Product Liability Directive. If the general-purpose AI model is put into service in the market regardless of whether it is made freely available or payable for a fee, Article 5 of the Product Liability Directive entitles a person who has suffered “damage” from a defective product to compensation.
“Damage” is defined in Article 6 of the Product Liability Directive and includes “death or personal injury, including medically recognized damage to psychological health” or “damage to, or destruction of, any property”.
Red teaming may have an impact on determining whether a general-purpose AI tool is deemed to be a “defective product” under the Product Liability Directive. If the manufacturer of the AI tool (in this case the developer of the AI tool or even a company that has provided the AI tool under its own trademark, even if it is not the developer of the said AI tool) has knowledge of the AI tool providing malicious output based on its red teaming activities, the AI tool may be assessed as “defective” pursuant to Article 7 of the Product Liability Directive.
Defectiveness could arise due to the manufacturer’s knowledge that there is a likelihood of the general-purpose AI tool providing malicious output if the manufacturer could reasonably foresee the AI tool being used by a consumer in a manner that would result in such malicious output being provided. Ignorance of possible malicious output due to failure to conduct red teaming may violate the EU AI Act, which under certain circumstances, requires providers of such AI tools to comply with red teaming requirements under the EU AI Act. An expanded discussion of this topic can be found below.
Ignorance of possible malicious output due to failure to conduct red teaming may violate the EU AI Act
In certain industries, internal IT teams may wish use to customize their AI tools to make its use more suitable for the industry by using retrieval augmented generation (RAG). RAG is “an AI framework that combines the strengths of traditional information retrieval systems (such as search and databases) with the capabilities of generative large language models (LLMs).” Companies may use their own data and combine it with pre-trained LLMs to create a general-purpose AI tool that fits its own needs.
There are several legal issues in relation to product liability when a company chooses to integrate a RAG with a pre-trained LLM. First, the developer of the pre-trained LLM may disclaim all liability of its LLM’s output if a RAG is integrated. This means that companies that develop a RAG should be aware of its liability exposure if it releases this AI tool (RAG + pre-trained LLM) for commercial use.
This does not only include liability from putting into the market a defective product, but also for any potential copyright infringement or any personal data transgressions. Furthermore, companies which integrate a RAG then become responsible for all red teaming activities to sift out malicious output. Red teaming costs should not be overlooked in such an instance.
EU AI Act
Under the EU Artificial Intelligence Act (EU AI Act) which was passed in 2024, providers as defined under the EU AI Act are specifically required to have “where applicable, a detailed description of the measures put in place for the purpose of conducting internal and/or external adversarial testing (for example, red teaming), model adaptations, including alignment and fine-tuning” if they are providing a general-purpose AI model with systemic risk. Article 55 sets out this obligation for providers of general-purpose AI models with systemic risks, which includes “conducting and documenting adversarial testing of the model with a view to identifying and mitigating systemic risks.”
Article 51 of the EU AI Act sets out what are the parameters which determine whether a general-purpose AI model is classified as one with a systemic risk. A model is classified as such if it has “high impact capabilities evaluated on the basis of appropriate technical tools and methodologies, including indicators and benchmarks.” It should be further noted that the EU AI Act provides the presumption that a general-purpose AI model is deemed to have high impact capabilities when the “cumulative amount of computation used for its training measured in floating point operations is greater than 10(^25).” A general-purpose AI model is also classified as one with a systemic risk if the EU Commission decides it to be so pursuant to Article 51(1)(b).
Crucially, providers under the EU AI Act are defined as “a natural or legal person, public authority, agency or other body that develops an AI system or a general-purpose AI model or that has an AI system or a general-purpose AI model developed and places it on the market or puts the AI system into service under its own name or trademark, whether for payment or free of charge.” A company which, for example, develops its own AI chatbot using models that are more than 10(^25) FLOPS and places it for use to the public would technically be required to comply with the provisions for a general-purpose AI that carries a systemic risk.
For the purposes of red teaming, such companies would, under the current interpretation of the EU AI Act, be required to conduct and detail experience with adversarial testing on their general-purpose AI model. From an EU AI Act compliance perspective, companies should in such cases consider enacting a red teaming policy and carve out a budget for red teaming as well.
In determining what is the standard of detail and quality required insofar where adversarial testing is concerned, the EU AI Act refers to “codes of practice” which should be published no later than May 2, 2025. Regardless, businesses aiming to launch general-purpose AI models should conduct red teaming and, additionally, keep a record of its red teaming activities.
Legal privilege
When red teaming is performed, a significant amount of information and details on the risks, processes, and procedures of the red teaming effort is documented. Such information is highly sensitive in nature, and in the case of potential litigation, claimants who claim to have suffered from the malicious output of a general-purpose AI model may request for the defendant’s red teaming activities.
While this may disincentivize or discourage companies from keeping a record of its red teaming efforts in a bid to reduce potential liability, companies should note that under the EU AI Act, documenting red teaming efforts is nonetheless a compliance requirement for general-purpose AI models with a systemic request.
Clients who are facing potential lawsuits in relation to AI tools should, prior to making any statement or providing information about its red teaming activities, seek counsel from their lawyers on the attorney-client privilege that may apply to the documentation surrounding its red teaming activities.
For instance, under Slovenian law, to determine whether legal privilege applies to a red teaming report and related documentation, the court will most likely consider whether the testing was performed in the scope of preparing legal advice about the system’s potential liabilities. While so far there is no case law in a similar scenario, it is possible that legal privilege could apply, especially if the results of the testing were adequately protected and treated as confidential in the counsel’s files. There is, however, no guarantees.
In addition, as soon as third parties are involved in the red teaming process, the plaintiff of the suit could go after them. Therefore, companies may wish to confirm the position of red teaming documentation vis-à-vis attorney-client privilege before conducting such tests, especially if it is reasonably foreseeable that the red teaming documentation would be subject to discovery or as evidence in a case.
Conclusions
Red teaming is an important facet of general-purpose AI tools and should not be ignored. Generally, companies that seek to modify pre-trained LLMs (for example with a RAG) and put such AI tools into use should consider if they are required to conduct red teaming activities and allocate sufficient resources for such activities. While red teaming activities can be outsourced to third parties, companies should still bear in mind responsibilities in managing third parties, confidential information, and ensuring that the general-purpose AI tool is sufficiently tested to prevent malicious output as much as possible.
Irene Ng Šega is Head of the APAC Desk and Senior Attorney (Singapore, New York) for the Corporate/M&A practice area at CMS. Saša Sodja is a partner and head of the corporate / M&A practice in CMS office in Ljubljana.
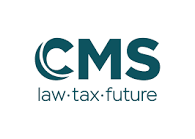