The Bank of England has published a speech given by Jonathan Hall, external member of the Financial Policy Committee, on the impact of AI developments on financial stability. The speech focuses on a subset of AI called deep learning (a form of machine learning where neural networks are trained on large amounts of data).
Hall discusses areas such as model failure and model misspecification, arguing that the financial market can be seen as an Information Processing System. The speech will be of interest to market intermediaries considering AI and machine learning (ML), repeating many key themes and concerns raised by regulators before in relation to appropriate governance and oversight arrangements for sign off and deployment and use of AI, as well as the need to have adequate systems in place for monitoring output.
What’s the current situation?
The increase in the use of electronic trading platforms and available data has led firms to consider the use of AI and ML in trading. According to the speech, there are performance management concerns about AI, and many in the market seem to be using ML mostly in supervised learning scenarios and not neural networks.
However, Hall considers a scenario where neural networks could become deep trading agents, selecting and executing trading strategies and the implications for market stability. He highlights two main risks:
- Deep trading agents could lead to an increasingly brittle and highly correlated financial market.
- The misalignment of incentives of deep trading agents with that of regulators and the public good.
Hall examines two types of trading algorithms, affectionally named Val and Flo: a value trading algorithm (an algorithm attempting to profit from an understanding of moves in fair value); and a flow-analysis trading algorithm (an algorithm attempting to understand and benefit from supply-demand dynamics).
In relation to deep value trading algorithms, Hall notes that volatile or unpredictable behavior can result if there are flaws in the training process, while sudden changes in the environment can induce failure. To achieve predictability and adaptability in a trading algorithm usually requires either extensive training or other predictability use techniques. Therefore, in the immediate term, internal stop limits and external human oversight (including kill switches) are needed to mitigate such unwanted volatility in trading algorithms, just as with such risks with a human trading desk.
Managers implementing highly complex trading engines must also have sufficient understanding that goes further than a single, simplified interpretation of the model.
In respect of flow analysis trading, Hall argues that the algorithm could see the potential that market instability offers for outsized profits and might be incentivized to amplify shocks. He argues that this could be addressed via training to respect rules or a constitution.
So what could be done?
There are three main areas of focus going forward, according to Hall:
- Training monitoring and control: Deep trading algorithms need to be trained extensively, tested in multi-agent sandbox environments and constrained by risk and stop loss limits. Managers need to monitor output for signs of unusual and erratic behaviour. The FPC needs to understand and monitor stability implications of any changes in the market ecosystem.
- Alignment with regulations: Deep trading algorithms need be trained in a manner that aligns with the regulatory rulebook. Training needs to be updated to respond to keep up with identified divergences between regulatory intent and reaction function, while trading managers need to keep reinforcing rules to ensure that they are not forgotten.
- Stress testing: Stress scenarios need to be based on adversarial techniques and not neural networks behaving in a smooth manner. Stress tests will need to understand the reaction function of deep trading algorithms, as well as be able to check performance and solvency.
How does this fit in with what is going on with AI so far?
AI has been on the regulatory radar internationally and at the UK level for some time. UK regulators published a Feedback Statement (FS2/23) in November 2023 (see our briefing Artificial Intelligence: UK regulators publish Feedback Statement and on GRIP Industry feedback on AI in financial services published ) to follow up on their joint 2022 discussion paper (DP5/22).
More recently, the BoE and the FCA published their approaches to applying the Government’s AI Regulatory principles: (1) safety, security, and robustness; (2) appropriate transparency and explainability; (3) fairness; (4) accountability and governance; and (5) redress.
The PRA and FCA take a technology neutral approach to regulation, but have flagged key areas of risk and use in the financial services sector (for example for trading/brokering clients in relation to market surveillance, trading bot software). Accountability and governance considerations have focused on the allocation of responsibility under the SMCR (SMF 24 in most cases, as opposed to a dedicated SMF for AI).
In its update, the FCA also sets out how its regulatory approach to consumer protection, particularly the Consumer Duty, are relevant to the fairness principle. At the international level, IOSCO guidance on AI and ML has stressed the importance of adequate testing and monitoring of algorithms to validate the results of an AI and ML technique on a continuous basis, as well as having appropriate controls in place.
Tim Cant, Lorraine Johnston, Jake Green, Etay Katz and Bradley Rice are partners in the financial regulation practice at Ashurt.
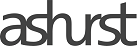